How to Use AI to Trade Stocks? As technology continues to advance, the intersection of AI and stock trading has become a topic of immense interest for both seasoned traders and those looking to explore innovative investment approaches.
This comprehensive guide will delve into the fascinating realm of AI-driven stock trading, exploring its underlying concepts, practical applications, and the potential benefits and risks associated with this cutting-edge technology. Whether you’re a seasoned investor seeking to enhance your trading strategies or a newcomer intrigued by the possibilities of AI in finance, this guide will provide valuable insights and actionable advice to help you navigate this exciting and rapidly evolving landscape.
Understanding AI in Stock Trading: Concepts and Terminology
Before diving into the intricacies of using AI for stock trading, it’s essential to grasp the fundamental concepts and terminology surrounding this powerful technology. By building a solid foundation of knowledge, you’ll be better equipped to appreciate the transformative potential of AI in the financial markets.
What is Artificial Intelligence (AI)?
Artificial Intelligence (AI) refers to the development of computer systems capable of performing tasks that typically require human intelligence, such as learning, reasoning, problem-solving, and decision-making. AI systems can analyze vast amounts of data, identify patterns, and make predictions or recommendations based on that data.
Machine Learning and Deep Learning
Machine Learning (ML) and Deep Learning (DL) are subfields of AI that enable computer systems to learn and improve from experience without being explicitly programmed. These techniques involve training algorithms on large datasets, allowing them to recognize patterns, make predictions, and continuously refine their performance.
- Machine Learning: ML algorithms learn from data and make predictions or decisions without relying on predefined rules or programming. Common examples include regression, decision trees, and support vector machines.
- Deep Learning: DL is a subset of ML that utilizes artificial neural networks inspired by the human brain to learn and make decisions. These neural networks can process and learn from vast amounts of data, including images, text, and numerical data.
Natural Language Processing (NLP)
Natural Language Processing (NLP) is an AI technique that focuses on enabling computers to understand, interpret, and generate human language. NLP algorithms can analyze text data, such as news articles, social media posts, and financial reports, to extract valuable insights and sentiment analysis.
Reinforcement Learning
Reinforcement Learning is a type of ML where an AI agent learns to make decisions by interacting with an environment and receiving rewards or penalties based on its actions. This approach can be particularly useful for developing trading strategies that adapt to changing market conditions.
AI in Stock Trading
AI techniques, such as Machine Learning, Deep Learning, Natural Language Processing, and Reinforcement Learning, are increasingly being applied to stock trading. These technologies can analyze vast amounts of data, including historical market data, news, social media sentiment, and financial reports, to identify patterns, make predictions, and generate trading signals or strategies.
AI-Powered Trading Strategies: Exploring the Possibilities
The integration of AI into stock trading has opened up a world of possibilities, enabling traders and investors to leverage advanced analytical capabilities and data-driven insights. In this section, we’ll explore some of the most promising AI-powered trading strategies and their potential applications.
Quantitative Trading and Algorithmic Trading
Quantitative trading, also known as algorithmic trading, involves using mathematical models and computer programs to automate the trading process. AI algorithms can analyze vast amounts of market data, identify patterns, and execute trades based on predefined rules or learned strategies.
- AI-driven Trend Analysis: AI algorithms can analyze historical market data to identify trends, price patterns, and potential entry and exit points for trades. This approach can help traders capitalize on market movements and make informed decisions.
- Sentiment Analysis and News Trading: By leveraging Natural Language Processing (NLP) techniques, AI systems can analyze news articles, social media posts, and other textual data to gauge market sentiment and make trading decisions accordingly.
- Portfolio Optimization: AI algorithms can be used to optimize investment portfolios by analyzing risk-return profiles, diversification, and asset allocation strategies, ensuring that portfolios are tailored to individual investment goals and risk tolerance.
High-Frequency Trading (HFT)
High-Frequency Trading (HFT) involves executing a large number of trades in fractions of a second, taking advantage of minute price discrepancies in the market. AI and Machine Learning techniques can play a crucial role in developing HFT strategies by analyzing vast amounts of real-time data and identifying profitable trading opportunities faster than human traders.
- AI-driven Order Execution: AI algorithms can analyze market conditions, order flow, and liquidity to optimize order execution, minimizing slippage and maximizing returns in high-frequency trading environments.
- Adaptive Trading Strategies: Reinforcement Learning algorithms can be used to develop adaptive trading strategies that continuously learn and adjust based on changing market conditions and trading outcomes.
Robo-Advisory and Automated Investment Management
Robo-advisors and automated investment management platforms leverage AI and Machine Learning techniques to provide personalized investment advice and portfolio management services. These platforms can analyze individual investor profiles, risk tolerance, and financial goals to create and manage tailored investment portfolios.
- AI-driven Portfolio Construction: AI algorithms can analyze market data, asset classes, and risk factors to construct optimal investment portfolios that align with an investor’s objectives and risk profile.
- Automated Rebalancing and Optimization: AI systems can continuously monitor portfolios and automatically rebalance assets or make adjustments based on changing market conditions, ensuring that portfolios remain aligned with investment goals.
AI-Powered Market Forecasting and Risk Analysis
AI techniques can be applied to market forecasting and risk analysis, providing traders and investors with valuable insights and decision support tools.
- AI-driven Market Forecasting: By analyzing historical market data, economic indicators, and other relevant factors, AI algorithms can generate market forecasts, identify potential trends, and suggest trading opportunities.
- Risk Assessment and Management: AI systems can assess various risk factors, such as market volatility, credit risk, and liquidity risk, to help traders and investors manage and mitigate potential losses.
- Scenario Analysis and Stress Testing: AI algorithms can simulate different market scenarios and stress test investment portfolios or trading strategies, providing valuable insights into potential risks and opportunities.
Implementing AI in Stock Trading: Key Considerations
While the potential benefits of AI in stock trading are significant, it’s important to approach this technology with a strategic mindset and a thorough understanding of the challenges and considerations involved.
Data Quality and Preprocessing
AI algorithms rely heavily on the quality and quantity of data they are trained on. In the context of stock trading, access to high-quality historical market data, news, and other relevant data sources is crucial. Additionally, data preprocessing techniques, such as cleaning, normalization, and feature engineering, play a critical role in ensuring the accuracy and reliability of AI models.
Model Selection and Validation
Choosing the appropriate AI model or algorithm for a specific trading strategy is essential. Different models may be better suited for different tasks, such as pattern recognition, prediction, or optimization. Furthermore, rigorous model validation and testing procedures must be implemented to ensure the reliability and robustness of AI-powered trading strategies.
Overfitting and Generalization
One of the challenges in using AI for stock trading is the risk of overfitting, where a model performs well on historical data but fails to generalize to new, unseen data. Techniques such as cross-validation, regularization, and ensemble methods can help mitigate overfitting and improve the generalization capabilities of AI models.
Computational Resources and Infrastructure
Implementing AI-powered trading strategies often requires significant computational resources, including powerful hardware (e.g., GPUs, high-performance computing clusters) and specialized software frameworks. Building and maintaining the necessary infrastructure can be costly and may require expertise in areas such as cloud computing, distributed systems, and parallel processing.
Regulatory Compliance and Risk Management
The financial industry is heavily regulated, and traders and investors must ensure that their AI-powered trading strategies comply with relevant laws, regulations, and industry best practices. This includes implementing robust risk management frameworks, adhering to market conduct rules, and maintaining transparency and accountability in decision-making processes.
Continuous Learning and Adaptation
Financial markets are dynamic and constantly evolving, which means that AI models trained on historical data may become outdated or less effective over time. To maintain the effectiveness of AI-powered trading strategies, continuous learning and adaptation mechanisms must be incorporated, allowing models to update and adjust to changing market conditions and new data patterns.
Integrating AI with Traditional Trading Strategies
While AI offers powerful capabilities for stock trading, it’s important to recognize that traditional trading strategies and human expertise still play a crucial role. Integrating AI with established trading approaches can often yield the best results, leveraging the strengths of both human intuition and machine intelligence.
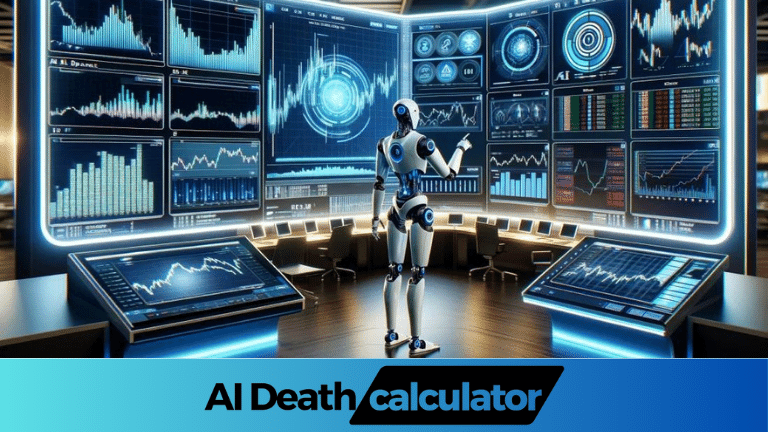
FAQs
What is AI trading, and how does it work?
AI trading, also known as algorithmic trading or automated trading, is the use of computer algorithms to make trading decisions. These algorithms analyze market data, such as price movements and trading volume, to identify trading opportunities and execute trades automatically.
How can I get started with AI trading?
To get started with AI trading, you can either develop your own trading algorithms or use existing AI trading platforms and software. If you’re new to AI trading, it may be helpful to start with a platform that offers pre-built algorithms and backtesting tools.
What are the benefits of using AI for stock trading?
Some benefits of using AI for stock trading include the ability to analyze large amounts of data quickly and efficiently, the ability to make trades automatically based on predefined criteria, and the potential to reduce human error and emotion in trading decisions.
What are some common AI trading strategies?
Common AI trading strategies include trend following, mean reversion, and momentum trading. Trend-following strategies aim to profit from the continuation of an existing trend, while mean reversion strategies aim to profit from the reversal of a trend. Momentum trading strategies aim to profit from the continuation of a trend.
Are there any risks associated with AI trading?
Like any form of trading, AI trading carries risks, including the risk of losses due to market volatility or algorithmic errors. It’s important to carefully evaluate the performance of any AI trading system before using it with real money and to have appropriate risk management strategies in place.